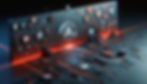
As digital landscapes expand and cyber threats grow more sophisticated, the conventional tools of cybersecurity increasingly fall short. Enter Artificial Intelligence (AI), a transformative force that’s redefining how we protect digital infrastructures and sensitive data. In this first part of our three-part series, we explore the integration of AI into cybersecurity, examining its roles, benefits, and the fundamental ways it enhances threat detection and management.
The Role of AI in Cybersecurity
AI in cybersecurity is not just an enhancement to existing systems; it’s a fundamental shift in how security protocols are designed and executed. AI technologies, especially machine learning and deep learning, are employed to create systems that can learn from data, recognize patterns, and make decisions with minimal human intervention. Here’s how AI is being utilized in cybersecurity:
1. Enhanced Threat Detection: AI systems are trained on vast datasets, which they analyze to detect anomalies and potential threats much faster than human analysts could. This rapid threat detection is crucial for preventing data breaches and system intrusions.
2. Behavioral Analytics for Security: AI excels in identifying subtle patterns in data. In cybersecurity, this capability is leveraged to monitor user behavior and network traffic, spotting unusual actions that could indicate a security threat, such as data theft or account compromise.
3. Automated Responses to Security Incidents: Once threats are identified, AI-driven systems can automatically take measures to mitigate damage, such as isolating affected systems, blocking suspicious IP addresses, and even initiating countermeasures against ongoing cyber attacks.
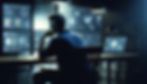
4. Predictive Capabilities and Proactive Security: By analyzing past and current data, AI models can predict future security incidents, allowing organizations to shift from a reactive to a proactive cybersecurity stance.
Understanding AI Technologies in Cybersecurity
To appreciate how AI transforms cybersecurity, it’s important to understand some of the key technologies involved:
- Machine Learning (ML): ML algorithms learn from historical data to identify patterns and make decisions. In cybersecurity, ML models are used to recognize the signs of potential threats, such as malware signatures or unusual network traffic.
- Deep Learning (DL): A subset of ML, deep learning uses neural networks with many layers (hence 'deep') to analyze various factors of data. DL is particularly effective in image recognition, natural language processing, and anomaly detection, which are pivotal in identifying sophisticated cyber threats.
- Natural Language Processing (NLP): AI’s ability to understand and interpret human language helps in automating threat detection from text-based data, such as emails and social media, to identify phishing attempts and other social engineering tactics.
Case Study: AI-Driven Intrusion Detection
Consider a global financial services firm that implemented an AI-driven intrusion detection system (IDS). The AI system was trained on years of security data, learning to differentiate between normal network behavior and potential threats. Within months, the system could identify threats with high accuracy, reducing false positives and allowing the security team to focus on genuine threats.
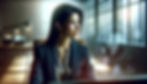
Advanced Threat Detection Using AI
AI enhances threat detection by integrating complex algorithms that analyze data at scale. Here’s how AI is applied in detecting various cyber threats:
1. Malware and Ransomware Detection: AI models are trained to scan code in applications and files, detecting malicious patterns and anomalies that signify malware or ransomware. For instance, AI-driven tools like Cylance use machine learning to predict and prevent executions of dangerous code without relying on signatures.
2. Phishing Attack Prevention: By analyzing the text and metadata of emails, AI can identify signs of phishing attempts, which often go unnoticed by traditional email filters. Tools like Barracuda Sentinel use AI to detect phishing schemes by looking for suspicious email patterns and intent.
Behavioral Analytics: AI’s Role in Understanding User Behavior
Behavioral analytics powered by AI plays a crucial role in identifying insider threats and compromised accounts. By continuously learning and profiling normal user behaviors, AI systems can spot deviations that may indicate a threat:
- User and Entity Behavior Analytics (UEBA): Products like Exabeam use machine learning to establish baselines of normal activity and alert on deviations, helping detect potential insider threats or compromised accounts.
AI in Incident Response and Automation
Rapid response to detected threats is crucial to minimize damage. AI significantly aids this process by automating responses:
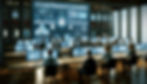
- Automated Incident Response: AI systems can automatically execute actions to contain and mitigate threats. For example, if an AI detects unusual outbound data traffic indicating a potential data breach, it can automatically block the traffic and alert administrators.
- Integration with Security Orchestration, Automation, and Response (SOAR): AI enhances SOAR capabilities to coordinate responses across different security tools. Palo Alto Networks uses AI to enrich SOAR platforms, streamlining security operations and response strategies.
Case Studies of AI in Action
1. Darktrace – AI for Real-Time Threat Detection and Response:
Darktrace uses AI algorithms to detect and respond to cyber threats in real time. Their systems model the ‘pattern of life’ for every device and user within a network and can spot subtle deviations that signal threats, effectively stopping them in real time.
2. Zscaler – AI and ML for Secure Internet and Cloud Access:
Zscaler employs machine learning to analyze billions of transactions, blocking attacks and preventing unauthorized access to internal applications, thus securing cloud environments.
Challenges of Implementing AI in Cybersecurity
While AI offers extensive benefits, its implementation is not devoid of challenges. Issues such as data privacy, AI bias, and the need for continuous training to keep up with new threats need to be addressed to leverage AI effectively.
AI's capabilities in cybersecurity are profound and transformative. By automating detection and response and providing deep insights into threats, AI tools are indispensable in the modern cybersecurity arsenal. In the final part of our series, we will explore future trends in AI-driven cybersecurity and the ethical considerations that come with it.
Having explored the roles and specific applications of AI in cybersecurity, we now turn our attention to the future. This concluding part of our series looks at emerging trends and the ethical challenges that AI brings to the cybersecurity landscape.
Emerging Trends in AI-Driven Cybersecurity
As technology evolves, so too do the capabilities of AI in enhancing cybersecurity defenses. Here are some key trends that are shaping the future:
1. Predictive Security: Leveraging big data and machine learning, cybersecurity systems are moving towards predictive models that can forecast attacks before they occur based on trends and anomalies detected across the global digital landscape.
2. Self-Healing Systems: AI is being integrated into systems that can not only detect and mitigate threats but also repair themselves automatically. This reduces downtime and enhances resilience against attacks.
3. Decentralized AI Security: With the rise of blockchain technology, decentralized AI security solutions are becoming more prevalent. These systems distribute the decision-making process, making it harder for attackers to exploit a single point of failure.
4. Integration of AI with Quantum Computing: As quantum computing becomes more accessible, its integration with AI will significantly enhance encryption methods and threat detection capabilities, setting a new standard in cybersecurity measures.
Ethical Considerations in AI-Driven Cybersecurity
With great power comes great responsibility. The deployment of AI in cybersecurity raises several ethical concerns that must be addressed:
- Privacy: AI systems often require extensive data to operate effectively, which can raise concerns about user privacy and data protection. Ensuring that these systems comply with data protection laws like GDPR is crucial.
- Bias and Fairness: AI models can inadvertently learn biases present in their training data. This can lead to unfair or discriminatory outcomes, such as incorrectly flagging activities from certain demographics as suspicious.
- Transparency and Accountability: There is a need for transparency in AI decisions, especially in a field as critical as cybersecurity. Users and regulators must understand how decisions are made to ensure accountability.
- Security of AI Systems: As AI systems become central to cybersecurity, the security of these systems themselves becomes critical. Protecting AI from attacks and ensuring that it cannot be manipulated by malicious actors is paramount.
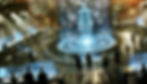
Looking Ahead
The future of cybersecurity is inextricably linked to AI. As we develop more advanced AI technologies, we must also be vigilant about securing these systems and ensuring they are used responsibly and ethically. Collaboration across industries and continuous dialogue on ethical AI use will be key to harnessing the full potential of AI in cybersecurity.
As we stand on the brink of what might well be described as a technological renaissance, the integration of Artificial Intelligence into cybersecurity represents a pivotal shift in our defensive paradigm. This series has traversed the expansive landscape of AI-driven cybersecurity, from its foundational roles and specific applications to the emerging trends and ethical considerations that shape its future. As we conclude, it is imperative to reflect on the broader implications of these advancements and the profound responsibilities they entail.
The promise of AI in cybersecurity is not merely about enhancing existing protocols or accelerating response times. It is about fundamentally transforming our approach to security from reactive to proactive, from isolated to interconnected, and from deterministic to predictive. AI’s ability to learn from patterns, anticipate threats, and adapt to evolving challenges presents an unprecedented opportunity to not only counteract but also foresee and forestall potential cyber threats.